FAQ’S, Dangers And Limitations Of Machine Learning
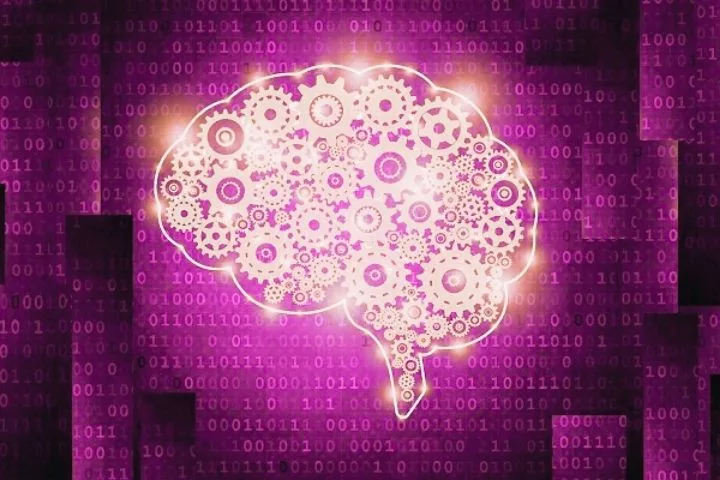
The dangers and limitations of machine learning
In addition to all these exciting applications and technological aspects. On the one hand, these dangers and limitations of machine learning relate to the fact that the result of the algorithm does not reflect reality. On the other hand, to excessive reliance on the results of a model.
BIAS
As noted several times, one of the main components in machine learning is the definition of the data to be used. Only when machine learning transforms data into knowledge is it possible to derive generalizations from it.
This harbors two dangers: On the one hand, there is the risk that the algorithm may be unconsciously influenced by choosing the wrong data. This is often the case when no user-centered research is carried out in advance, but the machine learning engineer defines the influencing data from his technical expertise.
Another factor is that only a specific range of data is available, but this does not necessarily have to be consistent with the highest causality.
The second danger is a bias due to the history of the data itself. If there is a very concise pattern in the data used, i.e., the past, this will also be found in the model, although this may not be intended.
The best example is the use of various recruiting algorithms in the FAANG environment. Neither Amazon nor Google manages to construct their machine learning-based CV evaluations free of sexism.
DATA QUALITY
As already mentioned in data cleaning, the data quality of the data used is fundamental for the quality of the output. Unfortunately, very often, data is incorrectly transformed, incorrect, or insufficiently documented. There is also often a lack of central data to give a model the informative power it deserves.
Consequently, one of the dangers is making decisions based on incorrect data and thus inaccurate models. This topic leads to the requirement of data governance & data management to form the basis for reliable machine learning implementations.
BLACK BOX
A frequently expressed criticism is that specific ML algorithms represent a black box. Especially in neural networks, due to the sheer variety of partial results (weights) and parameters, it is no longer understandable or only with immense effort, which is why a model makes a decision. This leads to the question of trust: Why does the machine learning algorithm make a decision?
To put it mildly, the black box metaphor is also relevant in today’s systems. The interpretability has improved, and more and more methods are emerging to define the inner workings of algorithms, but individual process steps are not always transparent.
But – and this criticism has to put up with this question – in many cases, an absolute understanding is unnecessary. As long as the data quality of the input is correct and the model is implemented correctly, only the output is essential – so the argumentation of some critics.
And this certainly applies to the majority of users, while other roles would like even more clarity about the processes and statuses.
OVERFITTING
Since machine learning modeling represents the optimization of a statistical model, an “optimal model” is usually specified as the goal. In supervised learning, the hyperparameters are generally adjusted until the lowest error rate can be observed in the test set.
While this gives the impression that the model depicts reality better and better, the opposite can be the case. This overfitting is the adaptation of the model to the training and test data until it optimally covers the available data but reacts poorly to new input. It is therefore essential to avoid overfitting while advancing the optimization.
INFLUENCE / DUNG TRAINING
Anyone who understands the processes behind machine learning can also conclude the algorithms implemented in a product. Consequently, it is also known how training and optimization work.
This also allows users to consciously influence the results. In the simple case, this is impacting Google search results or YouTube videos. This will enable users to control public effects in the more complex issue, as in the “Tay” case.
With the Tay chatbot, which communicated via Twitter, other Twitter users made Tay no longer speak like a teenager but like a racist and See. This happened through simple training and led to Microsoft switching off the bot. In summary, you have to think a lot about which interfaces and possibilities for influencing users are allowed.
Common questions about machine learning
WILL MACHINE LEARNING REPLACE HUMANS?
This is a much-discussed and more philosophical issue. In general, one can say that as long as there is no strong/general AI, the complexity of the human brain is not in danger. And even if there are, there are still many unanswered questions from learning to long-term planning to questions about hardware – many aspects that one could talk shop about.
Generally speaking, machine learning has so far not posed a threat to people and their work. Of course, some tasks will be automated, but of course, this is in the digital transformation.
The much more important aspect is that machine learning allows greater efficiency and accuracy and therefore improves one’s work. Thus, machine learning and other methods should not be seen as a danger or an opponent, but rather as a method and partner in the future.
HOW CAN I LEARN MACHINE LEARNING?
There are now various ways to learn machine learning or, as a higher-level topic, data science. From books to university degrees to countless online courses: there are many paths to the data.
Our recommendation, however, is to gain practical experience as early as possible so that you are confronted with real problems – such as poor data quality.
WHAT DOES A COMPANY NEED FOR MACHINE LEARNING?
Any company can gain initial experience with machine learning relatively quickly. Either by hiring a data scientist or hiring an external agency to implement the first use cases. However, these measures can only give a foretaste.
For the sustainable implementation and strategic use of machine learning, many aspects must be considered. From creating the organization, filling roles, opening up, and providing a range of data sources to data governance: machine learning modeling, especially in an agile way of working, requires a range of measures.
WHAT ARE THE ADVANTAGES OF MACHINE LEARNING FOR A COMPANY?
This is a comprehensive and highly individual question. Roughly speaking, machine learning can be applied in two categories: operational optimization or innovation. The former includes all of a company’s processes that are (partially) automated using AI or that identify additional information and patterns.
The second category is the use of data beyond that: either through the creation of new innovative work methods or even in the creation of new (digital) business models.
While not every company has the same benefits and limitations of machine learning, it will become increasingly mandatory to use this method in the coming years.
No matter which organization, no matter which company: More and more data about behavior, processes, and products are generated, and the evaluation is becoming increasingly complex.
Consequently, it makes sense for every company to think about the advantages machine learning can bring for their own company – and how these advantages can be developed.
IS AUTOMATED MACHINE LEARNING (AUTOML) THE FUTURE OF MACHINE LEARNING?
If we are talking about the entire limitations of machine learning or data science process, then certainly not. Shortly, automated machine learning tools will not be able to understand use cases, develop solution strategies, and interpersonal components of data relevance.
When it comes to the pure machine learning implementation and optimization process, there is a genuine chance that AutoML will soon at least be able to keep up with humans.
From feature engineering to model selection, AutoML platforms are often faster and more optimized than hand-written algorithms, which is why they are becoming increasingly attractive.